本文已被:浏览 440次 下载 952次 |
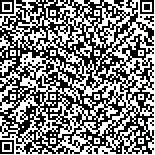 码上扫一扫! |
人工智能可解释性评估研究综述 |
李瑶,左兴权,王春露,黄海,张修建 |
|
(北京邮电大学网络空间安全学院,北京 100876;可信分布式计算与服务教育部重点实验室,北京 100876;;北京邮电大学计算机学院,北京 100876;北京航天计量测试技术研究所,北京 100076;国家市场监管重点实验室(人工智能计量测试与标准),北京 100076) |
|
摘要: |
近年来,可解释人工智能(XAI)发展迅速,成为当前人工智能领域的研究热点,已出现多种人工智能解释方法。如何量化评估XAI的可解释性以及解释方法的效果,对研究XAI具有重要意义。XAI的可解释性评估涉及主、客观因素,是一个复杂且有挑战性的工作。综述了XAI的可解释性评估方法,首先,介绍了XAI的可解释性及其评估的概念和分类;其次,总结和梳理了一些可解释性的特性;在此基础上,从可解释性评估方法和可解释性评估框架两方面,综述和分析了当前可解释性评估工作;最后,总结了当前人工智能可解释性评估研究的不足,并展望了其未来发展方向。 |
关键词: 可解释性评估 人工智能可解释性 主观评估 客观评估 评估方法 神经网络 深度学习 |
DOI: |
|
基金项目: |
|
Research Progress of Artificial Intelligence Interpretability Evaluation |
LI Yao,ZUO Xing-quan,WANG Chun-lu,HUANG Hai,ZHANG Xiu-jian |
(School of Cyberspace Security, Beijing University of Posts and Telecommunications, Beijing 100876, China;Key Laboratory of Trustworthy Distributed Computing and Service, Ministry of Education, Beijing 100876, China;;School of Computer Science, Beijing University of Posts and Telecommunications, Beijing 100876, China;Key Laboratory of Trustworthy Distributed Computing and Service, Ministry of Education, Beijing 100876, China;;Beijing Aerospace Institute for Metrology and Measurement Technology, Beijing 100076, China;Key Laboratory of Artificial Intelligence Measurement and Standards for State Market Regulation,Beijing 100076, China) |
Abstract: |
In recent years, explainable artificial intelligence(XAI) has developed rapidly and is becoming a research hotspot in the field of artificial intelligence. Many artificial intelligence explanation methods have emerged. How to quantitatively evaluate the interpretability of XAI and the effect of explanation methods are of great significance to the study of XAI. The evaluation of XAI's interpretability involves subjective and objective factors, which is a complex and challenging task. This paper reviews the interpretability evaluation methods of XAI. Firstly, the concepts and classifications of XAI's interpretability and its evaluation are introduced. Secondly, we summarize and sort out some characteristics of XAI's interpretability. On this basis, current studies on interpretability evaluation are reviewed and analyzed from both aspects of interpretability evaluation method and interpretable assessment framework. Finally, shortcomings of current studies on XAI's interpretability evaluation are summarized, and the future development directions are prospected. |
Key words: Interpretability evaluation Artificial intelligence interpretability Subjective evaluation Objective evaluation Evaluation method Neural network Deep learning |